
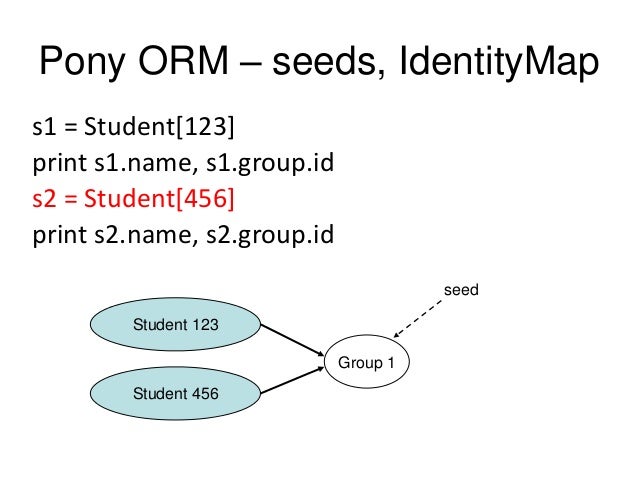
The following is the list of methods which can be used to generate datasets which could be used to train regression models. Methods for Generating Datasets for Regression Pipeline.score(X_test, y_test), pipeline.score(X_train, y_train) Pipeline = make_pipeline(StandardScaler(), LogisticRegression()) X_train, X_test, y_train, y_test = train_test_split(X, y, test_size=0.3, random_state=42, stratify=y) N_clusters_per_class=1, weights=, random_state=42) X, y = datasets.make_classification(n_samples=300, n_features=5, n_classes=2, n_redundant=0, # weights for each class (proportions of samples assigned to each class) # 200 records, 5 features, number of classes = 3 Here is a sample code.įrom sklearn.preprocessing import StandardScalerįrom sklearn.pipeline import make_pipelineįrom sklearn.model_selection import train_test_splitįrom sklearn.linear_model import LogisticRegression They are created in the order of informative first followed by redundant and then the repeated features. You can create features of three different types such as informative features ( n_informative), redundant features ( n_redundant) and duplicate features ( n_repeated).
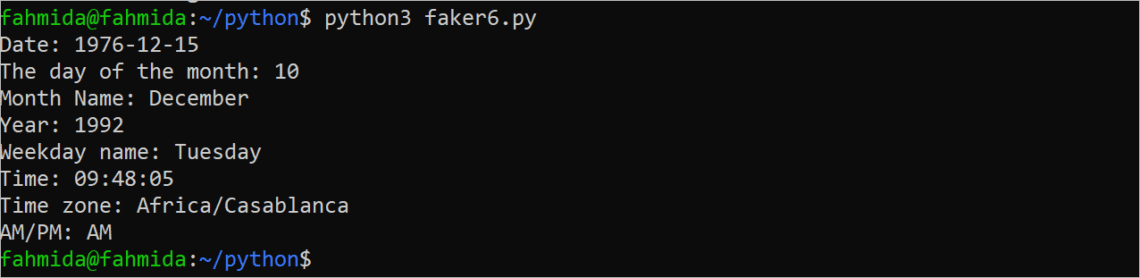
Other parameters that need to be carefully defined in case you have 3 or more classes / labels are weights and n_clusters_per_class. This dataset can have n number of samples specified by parameter n_samples, 2 or more number of features (unlike make_moons or make_circles) specified by n_features, and can be used to train model to classify dataset in 2 or more classes. make_classification: Sklearn.datasets make_classification method is used to generate random datasets which can be used to train classification model.Binary Classification Dataset using make_moons
